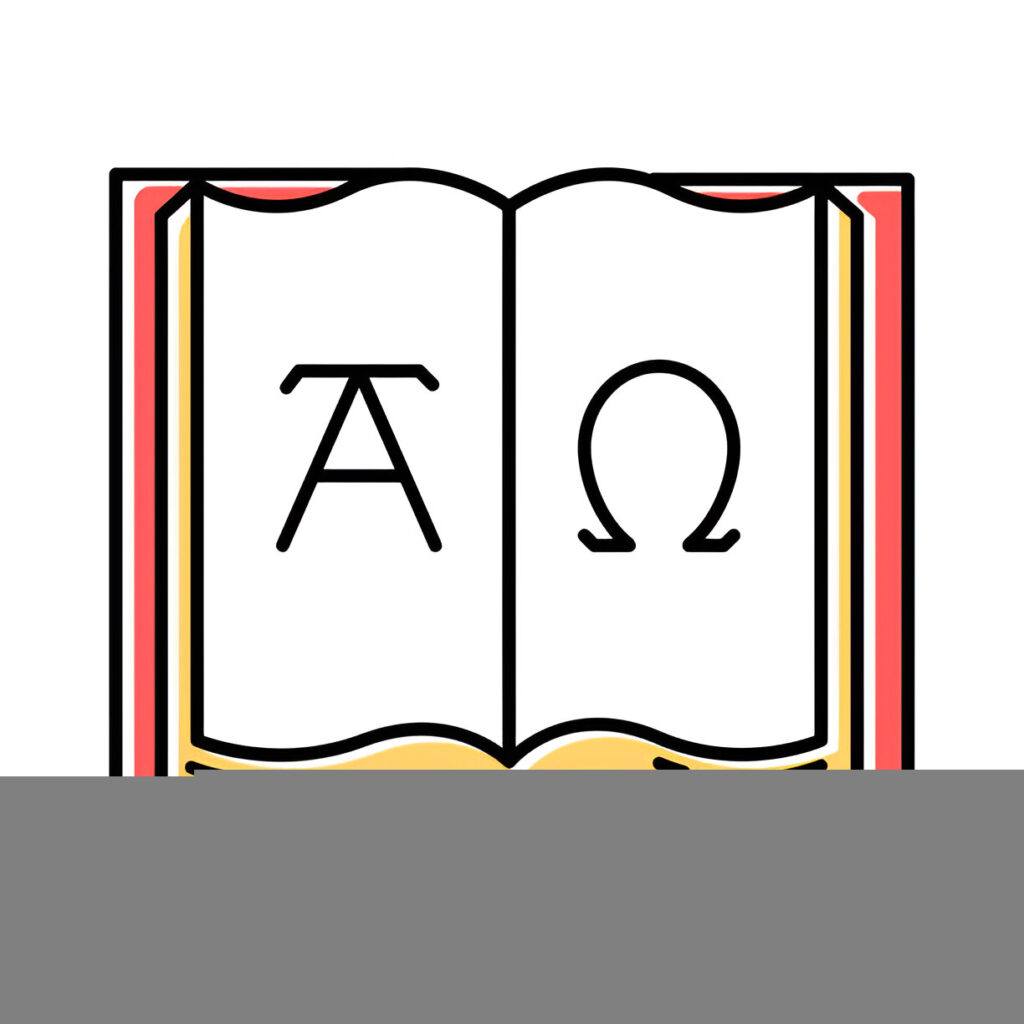
A Guide to Understanding AI Literature with John Ball’s Books
Artificial Intelligence (AI) has evolved from a speculative science into a transformative force reshaping industries, human behavior, and global decision-making. While tech giants dominate AI news cycles, deeper insights often emerge from thinkers and authors whose books dissect the philosophical, linguistic, and logical foundations of intelligent machines. Among such thinkers, John Ball has distinguished himself by approaching AI with a depth rooted in understanding human cognition itself. His literature not only critiques the shortcomings of current AI paradigms but also lays out an alternative vision that demands serious consideration.
John Ball’s contribution to AI literature isn’t just theoretical. His works—especially those detailing the Brain Simulator project—present a model of machine intelligence grounded in real-world understanding. Instead of relying on brute-force learning or statistical correlation, Ball’s approach emphasizes meaning-based learning and the replication of human cognitive processes. For readers unfamiliar with AI literature, his books provide a unique and accessible entry point that contrasts sharply with more technical or abstract works. They bring to the forefront questions about what intelligence really is—and whether current AI systems actually possess any.
The Need for Cognitive Clarity in AI
One of the fundamental problems in the AI field is the ambiguous use of the term “intelligence.” From autonomous vehicles to chatbots, systems are often labeled “intelligent” without clearly defining the criteria. This vagueness creates a landscape where real progress is hard to measure. John Ball’s writings challenge this ambiguity head-on. His books consistently ask: Can a system be intelligent if it doesn’t understand language, context, or causality?
This focus on meaning sets Ball apart. His argument rests on the idea that intelligence must be grounded in understanding. This involves knowing why something happens, not just that it happens. Ball often draws parallels between how humans acquire knowledge—from sensory inputs to language associations—and how AI systems should ideally be constructed. Instead of training models on massive data sets, he advocates for simulating cognitive functions, much like how a child learns about the world.
It’s this foundational view that differentiates Ball’s literature from the mainstream. While many authors and AI researchers discuss neural networks, deep learning, and reinforcement models, Ball emphasizes cognitive architecture. He suggests that unless machines are built to mirror how humans process concepts, they will never truly “understand” anything. This is particularly relevant in natural language processing (NLP), where understanding context, intent, and ambiguity remains a massive hurdle.
Rethinking Machine Intelligence
Books like “Conceptual Intelligence: The Path to Artificial General Intelligence” and “The Brain Simulator II” illustrate Ball’s model in detail. Rather than depend on probabilistic inferences, Ball proposes a concept-based model where relationships between objects, actions, and events form the core of intelligence. These relationships are not just stored but dynamically understood, allowing systems to adapt in real time.
Such an approach has profound implications. First, it challenges the assumption that more data equals better AI. Second, it highlights the limitations of black-box models like GPTs and convolutional neural networks. Ball argues that true AGI—Artificial General Intelligence—must emulate human-level understanding, which current architectures fail to achieve.
Moreover, Ball’s books serve as a practical guide for developers and researchers who want to build systems that think rather than react. He encourages readers to question the status quo and dig deeper into what intelligence entails. This makes his works both provocative and enlightening, especially for those growing disillusioned with the overhyped promises of mainstream AI.
Ball doesn’t just critique; he constructs. His Brain Simulator project, which is available as an open-source tool, serves as a testing ground for his theories. Readers of his books are often invited to explore this tool, giving them a hands-on experience of concept-based learning models. The simulator’s design aligns closely with his written theories, reinforcing the notion that understanding AI isn’t just academic—it’s also practical.
Diving into the Literature
Unlike most AI authors who inundate readers with equations, datasets, and code snippets, Ball writes for comprehension. His tone is engaging, his examples are rooted in everyday life, and his logical progression makes even complex ideas digestible. For someone new to AI or even a seasoned professional, reading Ball’s literature is like rediscovering the field from a completely different angle.
A common theme in his books is the inadequacy of current AI systems in dealing with context. For instance, while AI can translate languages or generate text, it often fails when asked to understand implications, sarcasm, or intent. Ball argues that this is because these systems are not actually “thinking”—they’re pattern matching. His literature points out that without a conceptual framework, these machines remain fundamentally unintelligent.
This distinction becomes clearer when examining how children learn. Children don’t memorize billions of sentences to understand a word. They associate the word with experiences, context, emotions, and outcomes. Ball insists that AI must follow a similar path if it is to progress from mimicry to actual intelligence. This childlike learning process, he believes, is the key to unlocking the full potential of AGI.
Within the scope of these discussions, the name John Ball Ai expert naturally emerges. His approach challenges prevailing trends and opens up alternative possibilities that are often ignored by mainstream academia. For readers seeking substance over hype, his perspective offers clarity and depth rarely found in AI literature.
One can appreciate how John Ball Ai expert brings a refreshing, almost philosophical edge to the field, one that prioritizes understanding over performance benchmarks. His insistence on meaning over data volume steers the conversation toward a more sustainable, ethical, and long-term vision for AI development. His literature does not only reflect a deep understanding of how the human brain functions but also lays out a framework for replicating that in machines—an audacious yet plausible goal.
A Framework Rooted in Common Sense
Another strong point in Ball’s books is the emphasis on common sense—something sorely lacking in today’s AI. Current models can outperform humans in chess and Go, yet they can’t tie shoelaces or understand the difference between a metaphor and a fact. Ball’s emphasis on replicating human understanding through structured, conceptual knowledge seeks to fill this gap.
He proposes a system where objects, actions, attributes, and emotions are interlinked in a vast network of associations. Each interaction updates this network, mirroring how human experience continually reshapes understanding. This model allows for flexibility, learning, and generalization—traits that current AI systems, despite their computational power, still lack.
This conceptual network can grasp that a cup can be used to drink water, hold pens, or even be thrown in anger. Such flexible understanding stems from knowing the why behind actions, not just the what. Ball’s literature repeatedly stresses that AGI must have this ability to generalize beyond training data and react sensibly to novel situations.
Impact and Practical Applications
While some may consider Ball’s approach more theoretical, his work is deeply practical. His Brain Simulator is used not just by enthusiasts but also by academic researchers and developers exploring alternative pathways to intelligence. It allows users to model conceptual learning systems, simulate conversations, and experiment with human-like understanding in a digital format.
Moreover, the ideas in his books are increasingly relevant in discussions around AI safety, interpretability, and ethics. By creating systems that understand context, intention, and consequence, developers can reduce the risks of AI misbehavior. Ball’s framework inherently supports explainable AI, because its reasoning is based on logical relationships, not hidden statistical weights.
Educators, policymakers, and researchers alike can benefit from reading Ball’s work. His books serve not just as a roadmap to building intelligent systems but also as a manifesto for a more thoughtful, responsible AI future. They prompt critical reflection on whether we are heading in the right direction—and what alternative paths might offer.
Final Thoughts
As AI continues to evolve at a breathtaking pace, the need for grounded, thoughtful perspectives has never been greater. In a world saturated with buzzwords and inflated claims, John Ball’s literature offers something rare: a genuinely new way to think about intelligence. His ideas are not just technical but philosophical, ethical, and deeply human.
Reading Ball’s books isn’t just an academic exercise; it’s a mental shift. They compel readers to question the assumptions behind modern AI, reconsider what it means to be intelligent, and explore new directions that could redefine the field entirely. Whether you’re an AI professional, a curious reader, or someone looking to make sense of the AI revolution, Ball’s works provide a vital lens.
In conclusion, understanding AI literature requires more than just familiarity with models and algorithms. It demands engagement with the ideas that challenge the status quo and expand our collective imagination. For that, few voices are as essential—or as compelling—as , whose contributions to AI literature continue to illuminate the path toward truly intelligent machines.